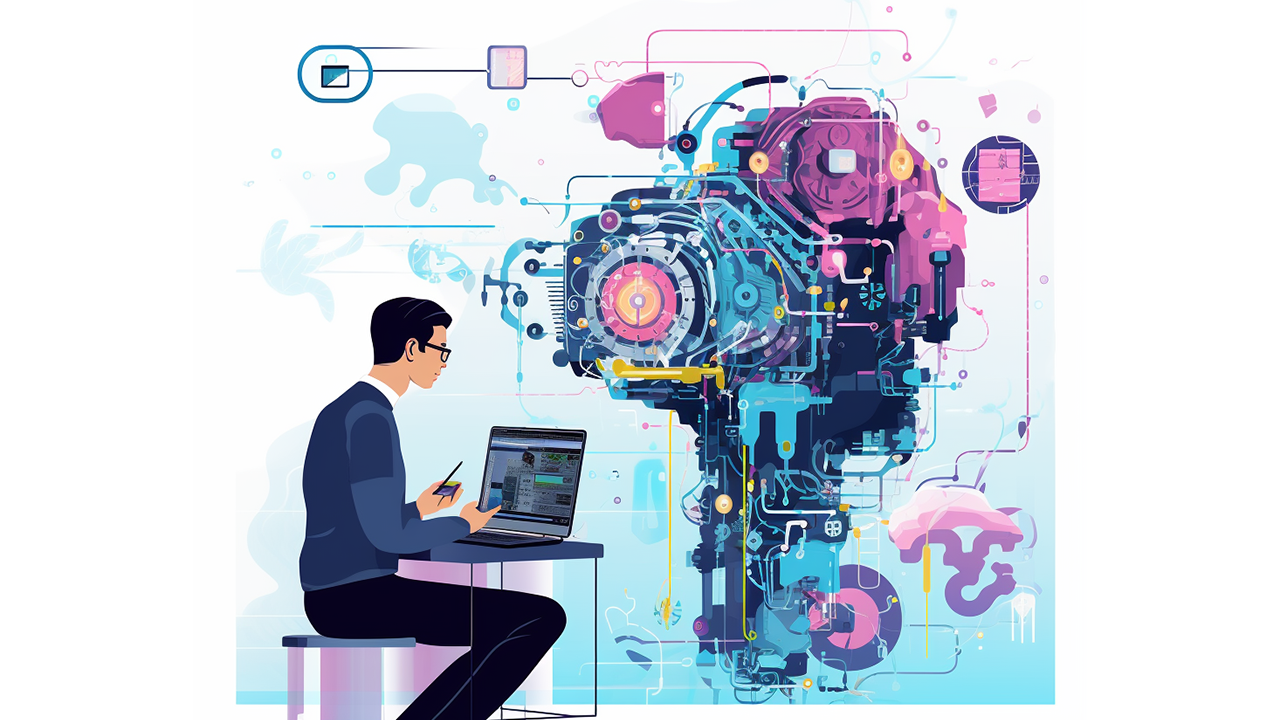
Enhancing AI with Retrieval-Augmented Generation for Precision and Relevance
Introduction
In the evolving landscape of artificial intelligence, keeping chatbots and digital assistants informed is crucial. Retrieval-Augmented Generation (RAG) is revolutionizing how we update these AI systems, providing them with the ability to offer precise, timely, and contextually relevant responses.
What is RAG?
Generative AI, with its core in Large Language Models (LLMs), has been exceptional in generating coherent text responses. However, it’s traditionally handcuffed by the data it was trained on, which could quickly become outdated. RAG liberates AI from these constraints by integrating up-to-date and specific data into the response generation process without the need to retrain the model1.
Application in Real-World Scenarios
Imagine a sports chatbot updated in real-time with player stats, game results, and expert analyses. RAG makes this possible by allowing access to a vast array of current data, from databases to live news feeds, ensuring that the information provided is both current and accurate.
Advantages of RAG over Traditional AI
RAG's dynamic nature means that it builds upon a knowledge repository that can be continually updated. This keeps the AI's responses fresh and context-aware, unlike the static nature of conventional AI models. The RAG framework thus significantly enhances the value of conversational AI systems.
How RAG Works
RAG functions by transforming varied data forms into a unified format that AI systems can understand. This data is then processed into numerical representations and stored efficiently, ready to be called upon when relevant queries are made.
RAG vs. Semantic Search
While semantic search seeks to understand query meanings deeply, RAG goes a step further by bringing in the dimension of continuous learning and data sourcing to improve accuracy and relevance over time.
Conclusion
RAG is setting a new standard for generative AI by offering an innovative solution that keeps conversational AI systems informed and intelligent. As this technology matures, it promises to bring more nuanced and sophisticated interactions between humans and AI.
Explore Further:
Interested in incorporating RAG into your AI solutions? For a detailed exploration and step-by-step guidance on RAG, check out Langchain's comprehensive documentation. It's an invaluable resource for those ready to enhance their AI systems with the power of real-time data retrieval. Dive into the world of advanced AI with Langchain's RAG resources here.
Popular Tags
Recent Posts
How To Choose The Right Software Development Company For IT Resource
ChatGPT for Commercial Use: How to Enhance Any Business With AI
Enhancing AI with Retrieval-Augmented Generation for Precision and Relevance
Smart Retailing with ChatGPT: 2023's Supply Chain Breakthroughs
We are at
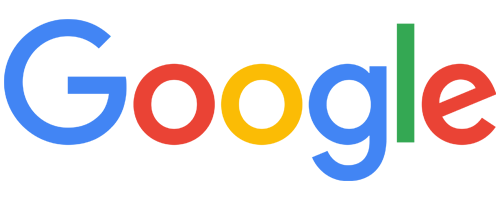
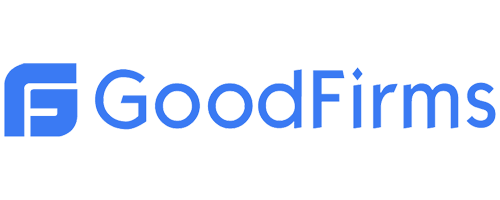
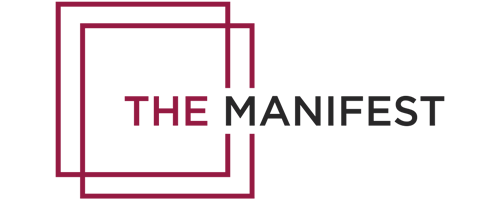
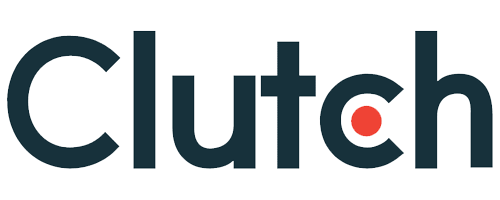
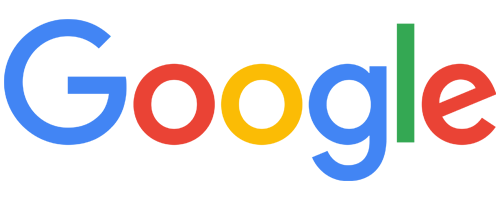
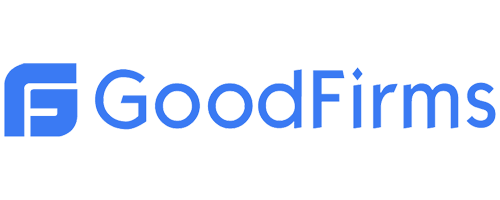
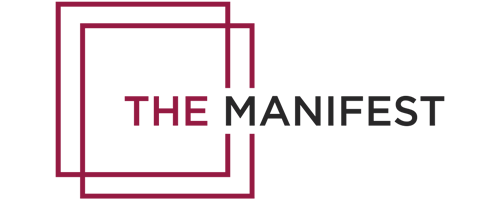
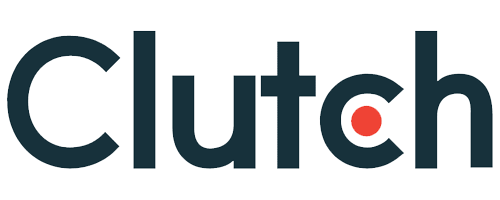
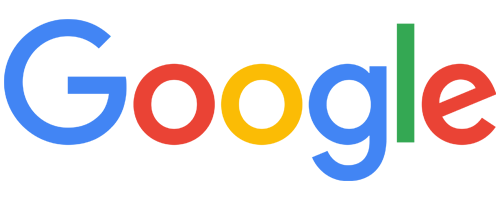
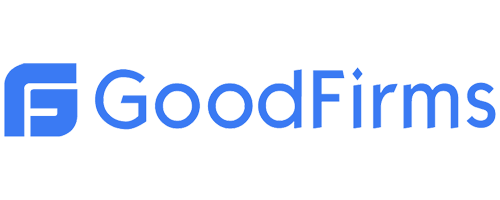
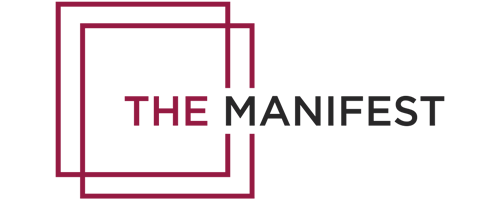
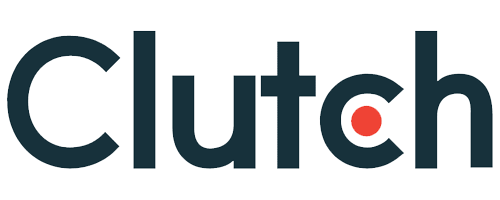
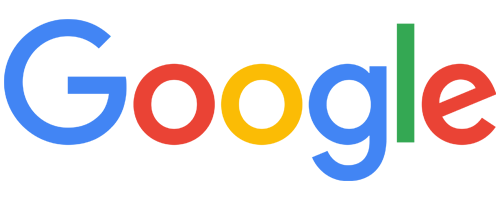
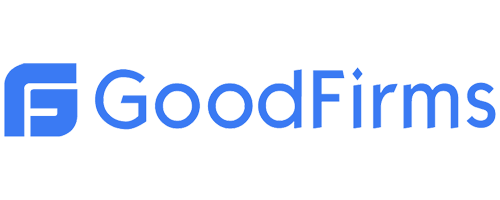
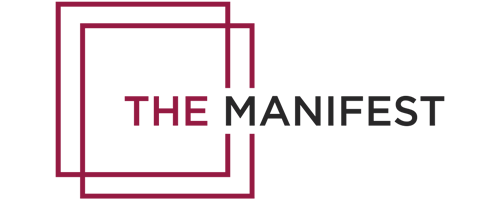
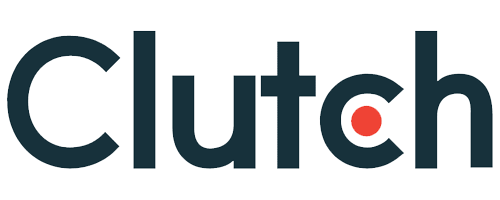
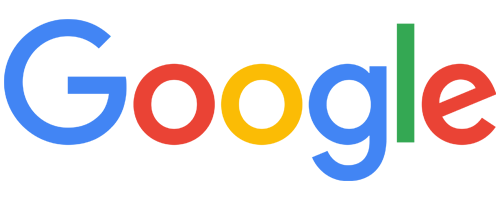
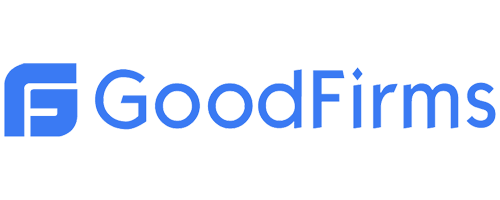
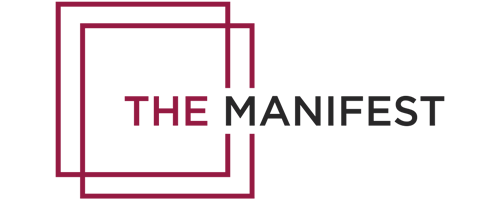
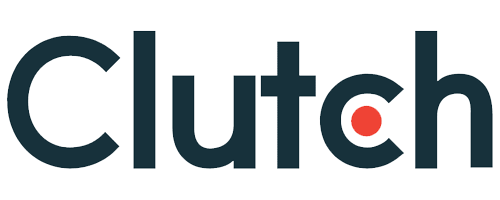